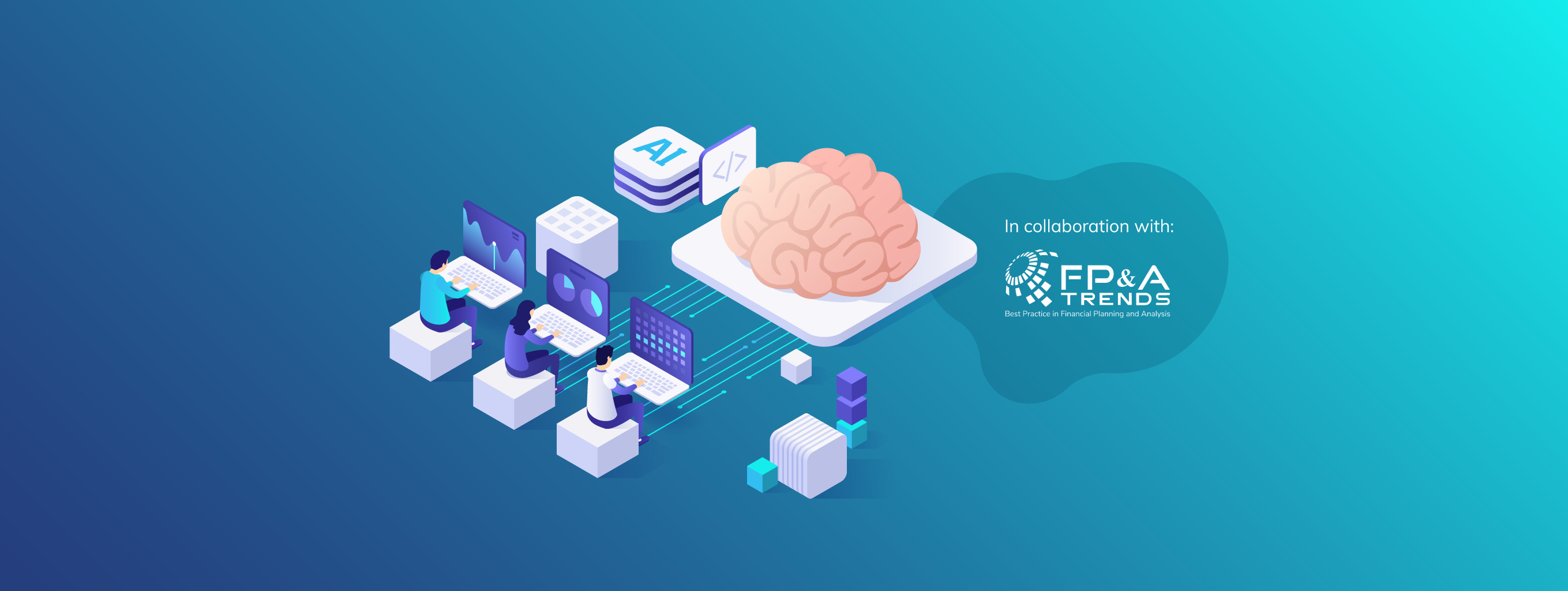
Combining AI insights and human expertise to transform FP&A
Organizations are increasingly turning to Artificial Intelligence (AI), Machine Learning (ML), and Generative AI (GenAI)…
AI/ML is increasingly part of our everyday lives. In the same way that mobile phones, the Internet, and apps have transformed communication and shopping habits, AI/ML is transforming the way organizations function, touching every business process along the way. That change includes FP&A and the role it plays in supporting management.
AI/ML differs from traditional analytical techniques by using algorithms that adapt and improve their performance over time as a result of increased data exposure, hence the term Machine Learning (ML). Algorithms can process vast amounts of data, way beyond the capabilities of spreadsheets, which greatly improves the detection of trends and relationships. These learnings are then automatically incorporated into subsequent analyses, from which predictions and recommendations are put forward to the decision-making process.
Our latest FP&A Trends Survey showed that while only 7% of organizations currently make use of AI/ML technologies, 54% plan to do so in the next few years. The reasons stated for future adoption include the significant benefits being derived from AI/ML, such as improved and quicker forecasts (61% rating their forecasts as ‘great or good’ compared to 38% among non-users) and the freeing up of FP&A time to concentrate on more high-value activities.
However, we believe these benefits are just the start of a more fundamental revolution that will redefine user experience and the role of FP&A.
Figure 1: Areas of AI impact in FP&A
It is worth mentioning that technology, in general, can greatly improve FP&A by eliminating manual and low-value tasks, such as collecting and validating data, as well as generating and distributing reports. However, the introduction of Generative AI and the use of ML will drastically change FP&A in the following areas:
We are all familiar with AI-powered ‘Chatbots’ that interpret text and voice queries to provide answers. They have become the norm for most customer-centric activities, such as call centers and price quotes. In the same way, Generative AI, through the use of natural language, is poised to revolutionize the way FP&A interacts with information and systems.
For example, on request or in response to an unexpected variance, it will autonomously gather data, conduct analyses, run scenarios, and present findings to the user, along with suggestions for actionable responses. Responses could also be automated on request, such as adjusting budgets or reallocating resources. As a result, FP&A’s traditional role in responding to inquiries and guiding the planning process will become obsolete.
Everyone we speak to starts their use of AI/ML to improve forecasting. By analyzing historical data, AI/ML can make more sophisticated predictions about the future. It can do this for each individual product or service and can model the detailed behavior of markets and customers by territory, as well as consolidate it all to provide a company-level forecast. To begin with, only one or two profit and loss (P&L) items are forecast for a particular region, but as the technology proves itself, the scope can be expanded to all of the major P&L categories across the whole company.
When outputs are automatically transferred to driver-based models, the time-saving can be substantial. Scott Campbell, Head of Digital COE – Finance / FP&A at Philips, explains that with this approach, their team can run a new forecast and automatically generate 80% of the complete P&L within 3 hours of receiving a new set of sales figures. Previously, this would have taken several days and involved 200 people. Overall, they found Machine Learning produces predictions that are 1.6% more accurate in predicting actual results than their previous business predictions.
Closely connected to improved forecasting is AI/ML’s ability to identify the factors that directly impact performance, in other words, the drivers. Drivers can be both internal, such as production capacity, and external to the company, such as the price of raw materials and competitor pricing. Detecting these relationships with conventional tools is often difficult and can take a considerable amount of time due to the size and complexity of the data involved.
There are different categories of algorithms within AI/ML that can be used to detect patterns and relationships in a wide variety of data. These can then be employed by predictive algorithms to forecast potential future driver values.
By utilizing different types of algorithms, organizations can quickly spot emerging trends and their potential impact on future performance.
There are many challenges organizations face when adopting AI/ML. These include:
Most, if not all, of these challenges can be solved by creating a pilot project to assess and learn how AI/ML can be used within the organization. For one company we spoke with, this meant educating the finance team on the technology and hiring an external consultant to implement a pilot project. The results were then used to improve the organization’s level of AI/ML maturity.
To sum up, AI/ML will transform FP&A into a better Business Partner. It will not replace the FP&A function as there are many things that the technology cannot do, such as think, and it will not always possess the data necessary to provide the basis for informed decisions. Similarly, AI/ML does not fully comprehend what makes a decision ‘good’ or ‘bad’ as not all objectives are quantifiable. However, the technology will fundamentally shift the role of FP&A, as explained above.
This transformation will require organizations to:
To help with this transition, we recommend reading the latest FP&A Board Maturity Model report.
How are finance leaders mastering leadership, skills, and business partnering?